Do you scour the internet for 'random forest dissertation'? Here you can find questions and answers on this topic.
The first part of this dissertation demonstrates that a haphazard forest is A fruitful framework stylish which to cogitation AdaBoost and esoteric neural networks. The work explores the concept and secondary of interpolation, the ability of A classifier to absolutely fit its education data.
Table of contents
- Random forest dissertation in 2021
- Random forest algorithm research paper
- Random forest theory
- Random forest generalization
- Random forest book pdf
- Random forest robust
- Understanding random forests: from theory to practice
- Random forest explained
Random forest dissertation in 2021

Random forest algorithm research paper

Random forest theory
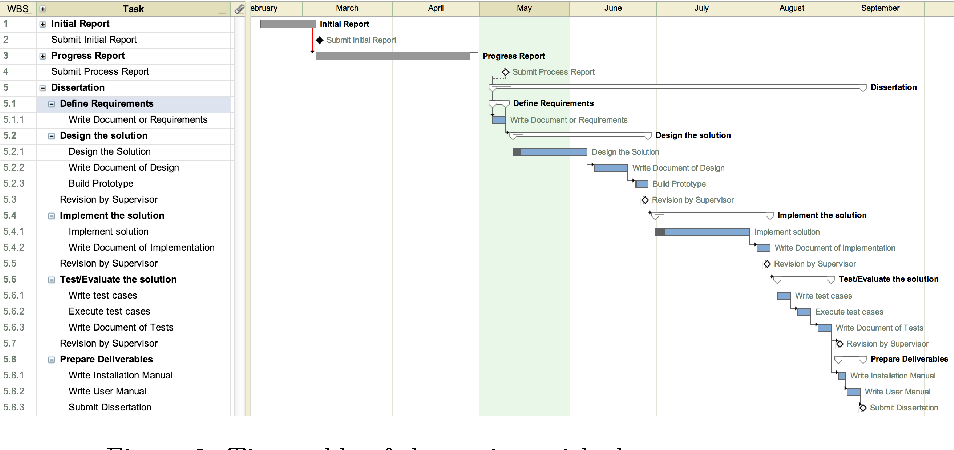
Random forest generalization
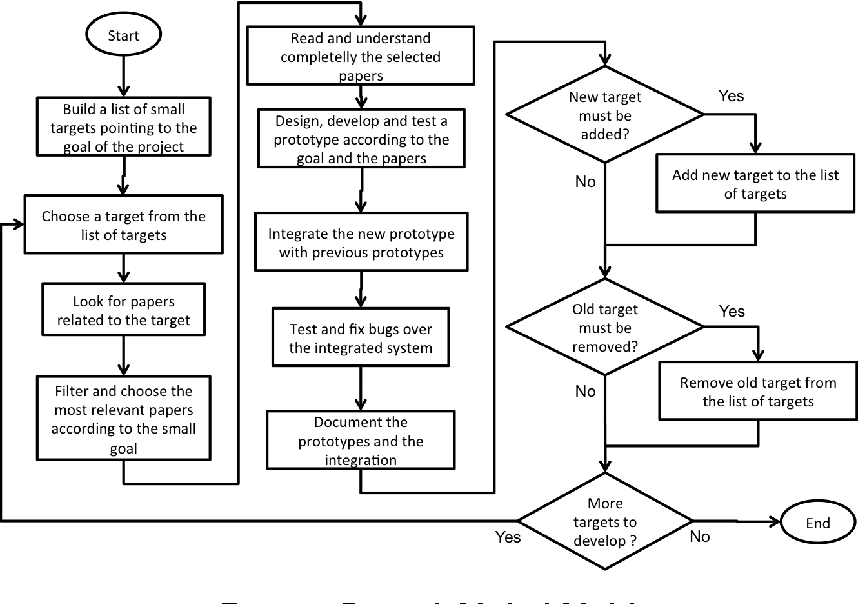
Random forest book pdf
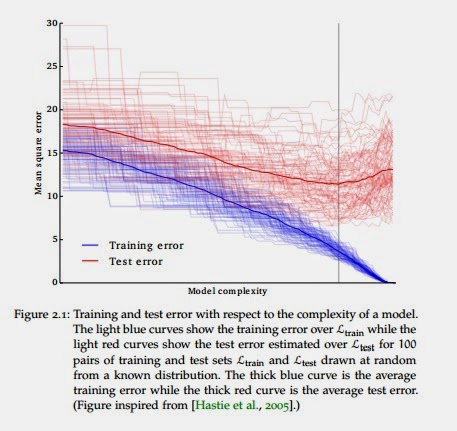
Random forest robust

Understanding random forests: from theory to practice

Random forest explained

What is the goal of a random forest thesis?
Accordingly, the goal of this thesis is to provide an in-depth analysis of random forests, consistently calling into question each and every part of the algorithm, in order to shed new light on its learning capabilities, inner workings and interpretability.
How is random forest used in data science?
They translate that data into practical insights for the organizations they work for. As a data scientist becomes more proficient, they’ll begin to understand how to pick the right algorithm for each problem. One extremely useful algorithm is Random Forest—an algorithm used for both classification and regression tasks. Confused?
How are decision trees trained in a random forest?
Decision trees in an ensemble, like the trees within a Random Forest, are usually trained using the “bagging” method. The “bagging” method is a type of ensemble machine learning algorithm called Bootstrap Aggregation.
Who are the authors of random forest robustness?
Random forest robustness, variable importance, and tree aggregation Graduate Theses and Dissertations Iowa State University Capstones, Theses and Dissertations 2018 Random forest robustness, variable importance, and tree aggregation Andrew Sage Iowa State University Follow this and additional works at:https://lib.dr.iastate.edu/etd
Last Update: Oct 2021